
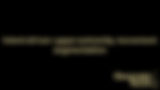
0.2s Speed
Human Intention Prediction
x 5 Stronger
900 N Force Support
Triple Motions
Bicep, Tricep, Shoulder
Upper-limb exoskeleton
Your Intention is all we need.
Bio-Interfaced Translational Nanoengineering Group
INTRODUCING
NEXT GENERATION OF EXOSKELETON.
Why Exoskeleton?
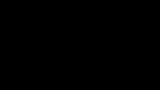
IoT-enabled upper-limb wearable exoskeleton with motion intention prediction.
Elderly patients, particularly the 20-40% of the 12.2 million annual global stroke victims with resultant neurological impairments and upper-body musculoskeletal weakness, face significant challenges in performing daily tasks independently.

Applications
Intent-driven Sensory System
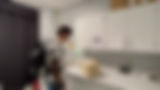
Exoskeleton's capability to augment strength for heavy objects We believe that the ideal exoskeleton robot for human strength augmentation should be able to (i) predict the user’s intended movement with high accuracy, (ii) be portable, (iii) be lightweight, (iv) be easy to use, (v) support the multiple upper-limb joint movements and (vi) incorporate the high-fidelity sensory feedback with motion-artifact-free and non-allergic EMG signal sensors. In this respect, to the authors’ best knowledge, there has been no work that totally integrated all the ideal elements into a fully functional exoskeleton that can assist individuals with the neuromotor disorder in performing everyday tasks in a completely user-friendly fashion.
A robotic exoskeleton that assists upper limb movements. It integrates sensory feedback, strength augmentation, and an algorithm predicting human intention in real time. EMG signals are wirelessly recorded via a microcontroller and Bluetooth system-on-chip, transmitting data to a mobile device using Bluetooth low energy. The system uses a deep-learning-based cloud computing platform, and the exoskeleton, powered by pneumatic artificial muscles, generates strong mechanical force to assist user movements. The exoskeleton's portable, lightweight design enhances user mobility while enabling natural movements. Additionally, a wireless, artificial EMG-sensing skin provides high-quality multi-channel electrophysiological signal monitoring for reliable sensory feedback.
Strength Augmentation for daily life
Experience Skin-Like wireless EMG sensor
Bio Translational Sensor with Electromyography (EMG) combine upper-limb joint movements enhance the functionality of the exoskeleton

Skin-like EMG sensor fabrication. the fabrication process of the proposed system involved several steps. In fabric substrate fabrication, Silbione parts were mixed and spin-coated onto a PTFE sheet. After curing, the PTFE sheet was detached, leaving a fabric substrate. Circuits and encapsulation were fabricated using a flexible PCB (fPCB) and electronic components mounted with reflow soldering. Laser cutting was performed to enhance mechanical flexibility. A lithium polymer battery assembly, elastomers, and encapsulation materials were used for power supply and protection. The nanomembrane electrode fabrication process involved depositing gold/chromium electrodes by E-beam evaporation and laser cutting. PDMS and a polymer film were used as layers, and gold was deposited and laser ablated to create the electrode pattern. Non-functional materials were removed, and the gold electrodes were transferred to the fabric using water-soluble tape. The fabric was laser cut, and the soft-packaged electronic system was attached to the fabric substrate using silicone.
Battery
Sensor: 6+ Hours
Motor: 8+ Hours
Response Rate
200-250ms
Weight
Total 4.7 KG
Location
Biceps brachii, Triceps brachii, Medial deltoid, and Latissimus dorsi
Connection Range
15-25m
The EMG data from multiple muscle locations were sent to Google Cloud using a custom Android app. The cloud architecture involved preprocessing the data and applying a CNN+LSTM algorithm developed with the Keras library in Python. The cloud returned real-time motion classes to the mobile device for exoskeleton driver actuation. The exoskeleton driver utilized a microcontroller, pressure feedback system, and valve control GPIOs to provide pressure control and actuate the exoskeleton based on the user's muscle motion signals. Deep-learning motion classification was performed using a CNN+LSTM model implemented in TensorFlow. The EMG signals were segmented and divided into training, validation, and testing sets. The model parameters were updated based on the validation accuracy, and hyperparameters were selected using a random search method. The best model was chosen based on validation accuracy and evaluated using the test dataset. The model utilized convolutional layers, convolutional cells, and fully connected layers to predict motion classes.


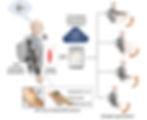
Specs

